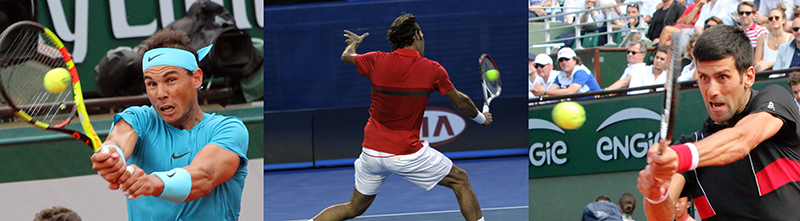
(L to R)Rafael Nadal, Roger Federer, and Novak Djokovic
The Australian Open ‘s 10,000-Point Hackathon Bears Fruit, But At Least One Fan Isn’t Sure
VIEW AND DOWNLOAD WORLD TENNIS GAZETTE VOL. 11 NO. 1
By JOHN MARTIN
March 2019
Melbourne was the seed bed but the entire planet was the garden where the ideas were expected to sprout.
So I was startled this winter when a team of data scholars from Queensland University of Technology–not quite 1100 miles away — announced they could tell me where Novak Djokovic was going to hit his next shot. Same with Rafael Nadal.
Roger Federer? Not so easy, they said from Brisbane. Turns out Federer is less predictable, said Dr. Simon Denman, a senior research fellow.
Rod Chester, a university writer, reported “The researchers analyzed more than 3400 shots for Djokovic, nearly 3500 shots for Nadal and almost 1900 shots by Federer, adding context for each shot such as whether it was a return, a winner or an error.”
Surprisingly, it didn’t take long.
“After about 1000 shots, the model (algorithm) has a pretty good idea of what is going on,” Dr. Denman said. “It needs about three matches to figure out a player’s style. Once it’s got those three matches it’s pretty solid.”
The data was drawn from the 2012 Australian Open, according to a story posted on the Internet.
“We had an analysis of how accurate it is for those three top players – Djokovic, Nadal and Federer,” Dr. Denman said.
“And it was least accurate who is perhaps the most versatile. It struggled the most to predict him. He can do anything, so the model was more often wrong about him.”
But the scholars did not entirely convince Chuck Wattles, a high school tennis coach in El Centro, CA. y.
An avid recreational player, Wattles observed, “If they thought Federer was hard to track, it’s a good thing they didn’t try to track my shots, (be) cause even I don’t know where they’re going.”
Two years ago, Australian tennis officials launched a massive survey of tennis match data they had collected from earlier Australian Opens. They announced they were focusing on forced and unforced errors recorded in hundreds of Grand Slam singles and doubles battles.
More than a dozen data scientists soon began guiding a “hackathon,” a global competition between some 700 entrants who examined digital clues from roughly 10,000 points played over the equivalent of a single Grand Slam championship.
They created spreadsheets from data generated by both men’s and women’s singles, according to Dr. Stephanie Kovalchik, a transplanted American data scientist who helped lead the Australian team.
During the 2018 Australian Open, she stood working at a computer console on the eighth floor of Tennis Australia’s gleaming new headquarters, which sits at the edge of Melbourne Park’s sprawling tennis complex.
Behind her, half a dozen analysts sat at desktop computers, mostly in silence as they examined and puzzled over data.
“It’s an exciting time for tennis, how we use data, and how we use it to predict outcomes,” said Craig Tiley, the Australian Open’s tournament director.
Tiley is a former tour-level player, successful American college tennis coach (NCAA Men’s Team Championship at the University of Illinois}, and a longtime student of statistics,
Nevertheless, as the Hackathon proceeded, Tiley conceded that he wasn’t certain where the Big Data search would lead.
“Even though I did statistics in college for four years, and I understand, you know, the regression analysis that you need to do,” he said, “but I don’t know the answer to that,” Tiley said.
Asked if his Game Insight Group’s work was intended to find ways to replace court statisticians and lines people with machines, Tiley said it was not.
Then he added, “I do think the future is going to involve, you know, line calling that’ll be automated, and data collection and analytics that’ll be auto mated, and a lot more.
Are shot predicting algorithms next? Will every coach want one? It’s hard to predict.